ConGeo: Robust Cross-View Geo-Localization Across Ground View Variations
Sep 29, 2024·,
,,,,·
0 min read
Li Mi
Chang Xu

Javiera Castillo Navarro
Syrielle Montariol
Wen Yang
Antoine Bosselut
Devis Tuia
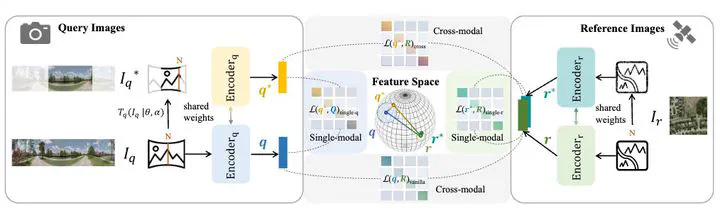
Abstract
Cross-view geo-localization aims at localizing a ground-level query image by matching it to its corresponding geo-referenced aerial view. In real-world scenarios, the task requires accommodating diverse ground images captured by users with varying orientations and reduced field of views (FoVs). However, existing learning pipelines are orientation-specific or FoV-specific, demanding separate model training for different ground view variations. Such models heavily depend on the North-aligned spatial correspondence and predefined FoVs in the training data, compromising their robustness across different settings. To tackle this challenge, we propose ConGeo, a single- and cross-view Contrastive method for Geo-localization: it enhances robustness and consistency in feature representations to improve a model’s invariance to orientation and its resilience to FoV variations, by enforcing proximity between ground view variations of the same location. As a generic learning objective for cross-view geo-localization, when integrated into state-of-the-art pipelines, ConGeo significantly boosts the performance of three base models on four geo-localization benchmarks for diverse ground view variations and outperforms competing methods that train separate models for each ground view variation.
Type
Publication
European Conference on Computer Vision (ECCV)